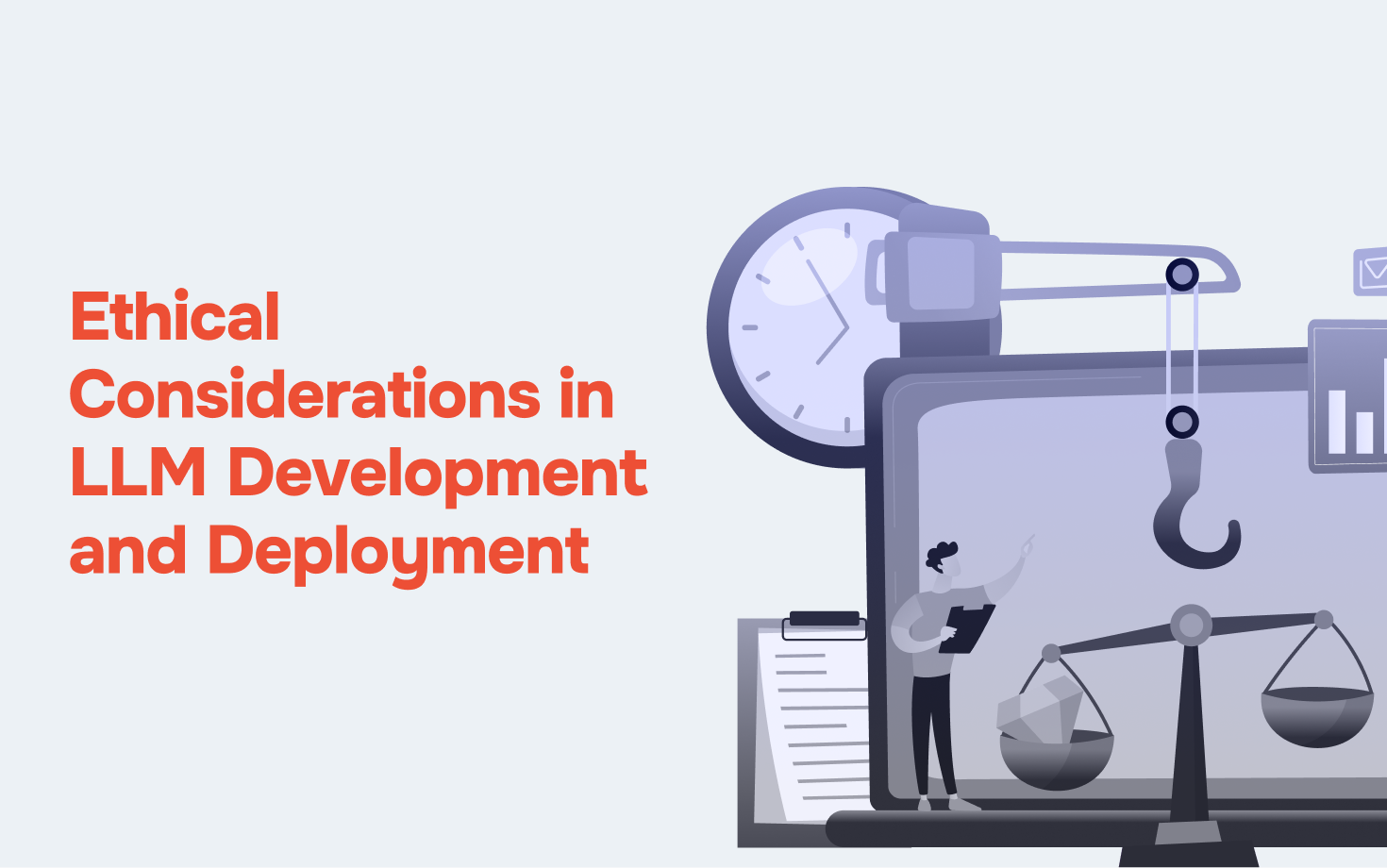
1. Bias in Training Data
One of the most significant ethical concerns in language model development is bias in training data. Language models learn from large datasets, and if these datasets contain biases, the models will replicate and potentially amplify them. Biases can be based on race, gender, ethnicity, religion, sexual orientation, and other factors. A model trained on internet text may inadvertently learn and reproduce stereotypes. To mitigate this issue, developers must carefully curate and preprocess training data to remove biases. Additionally, they can implement techniques such as data augmentation and adversarial training to make models more robust to biased inputs.
2. Language models learn from large datasets
Language models have the capability to generate text that can be harmful or offensive. For instance, they could produce hate speech, misinformation, or inappropriate content. This poses a serious ethical dilemma, as deploying such models could lead to real-world harm.
To address this concern, developers can implement filtering mechanisms to detect and prevent the generation of harmful content. This can involve using profanity filters, sentiment analysis, and human review processes. Clear guidelines should be established for what constitutes acceptable content.
3. Privacy and Data Security
Language models often require vast amounts of data to train effectively. This data may include personal information, such as emails, chat logs, or social media posts. There is a risk of privacy breaches if this data is not handled securely.
Developers must prioritize privacy and data security throughout the development and deployment process. This includes implementing robust encryption protocols, anonymizing data, and obtaining explicit consent from users before collecting their data.
4. Accessibility and Inclusivity
Language models should be accessible to everyone, regardless of their linguistic or cognitive abilities. However, many current models struggle with understanding and generating text in languages other than English or for users with disabilities.
Developers need to invest in research and development efforts to make language models more inclusive and accessible. This involves training models on diverse languages and dialects, as well as improving support for assistive technologies such as screen readers.
5. Accountability and Transparency
Developers should be transparent about how their models work, including the data used for training and the algorithms employed. Users should have clear information about the limitations and potential biases of these models. They must be accountable for the consequences of deploying language models. This includes establishing mechanisms for handling complaints and addressing instances of harm caused by the models.
How Hexon Global can help you with AI ?
Count on Hexon Global’s AI expertise and hands-on experience in deploying, managing, and fine-tuning AI and ML infrastructures on AWS. We ensure optimal performance and efficiency to meet the demands of your AI and ML workloads, while accounting for all potential biases and vulnerabilities.
Make Hexon Global your trusted ally in advancing your AI and ML initiatives on AWS. Reach out to us.
Contact Us
Get in touch
Understand how we help our clients in various situations and how we can be of service to you!