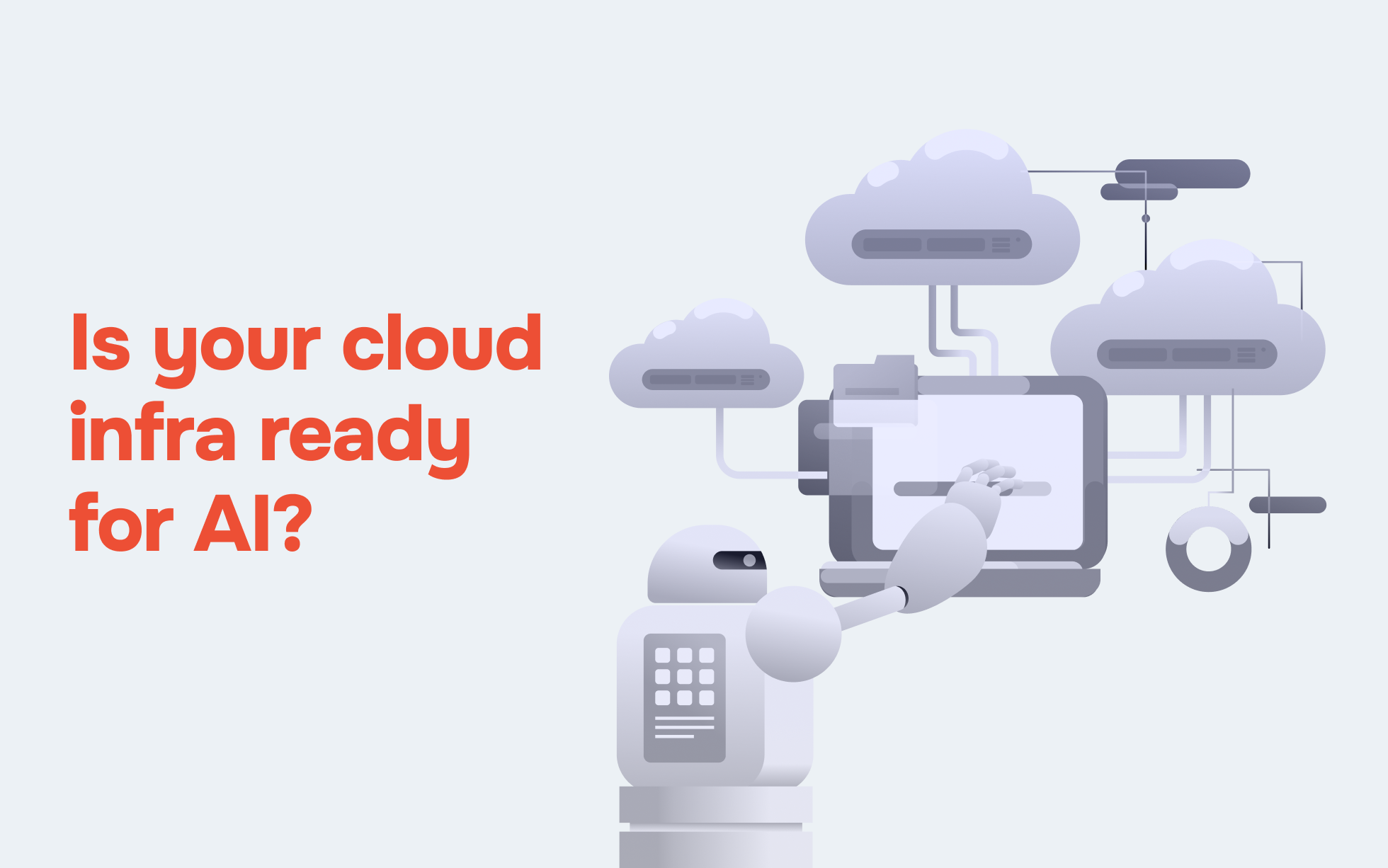
Understanding Advanced Language Models
Advanced language models are built on large-scale transformer architectures. These models are trained on massive datasets, comprising texts from the internet, books, articles, and other sources. Through this training, they learn to understand the nuances of human language, including grammar, semantics, context, and even cultural references.
The hallmark of these models is their ability to generate coherent and contextually relevant text given a prompt. This capability has far-reaching implications, from assisting writers and developers to automating content creation, and from improving chatbots to aiding in educational tools.
The Need for Cloud Infrastructure
The computational requirements for training and deploying advanced language models are immense. These models consist of billions or even trillions of parameters, and training them requires vast computational power and storage resources. Here’s where cloud infrastructure comes into play:
1. Scalability
Cloud platforms provide scalable resources, allowing users to scale up or down based on their computational needs. Whether it’s training a new model or deploying existing ones, cloud infrastructure ensures that the necessary resources are available when needed.
2. Compute Power
Training advanced language models involves complex mathematical computations, particularly matrix multiplications and optimizations. Cloud providers offer high-performance computing (HPC) resources, such as GPUs (Graphics Processing Units) and TPUs (Tensor Processing Units), optimized for these tasks.
3. Storage
The datasets used for training these models are vast, often ranging from hundreds of gigabytes to terabytes. Cloud storage solutions offer cost-effective and scalable options for storing these datasets securely.
4. Cost Efficiency
Cloud infrastructure allows users to pay only for the resources they use, avoiding the need for upfront investment in hardware. Additionally, cloud providers often offer pricing models tailored to AI workloads, making it cost-efficient for businesses and researchers.
5. Accessibility
Cloud platforms provide accessibility from anywhere with an internet connection. This allows distributed teams to collaborate on model development and deployment seamlessly.
Challenges of Using Cloud For Language Models
There are challenges in leveraging cloud infrastructure for advanced language models:
1. Cost Management
Training large models can incur significant costs. Proper resource management and optimization strategies are essential to control costs. Techniques like model distillation, where smaller models are trained to mimic the behavior of larger ones, can help reduce computational requirements.
2. Data Security and Privacy
Handling sensitive data raises concerns about security and privacy. Cloud providers offer robust security measures, including data encryption, access controls, and compliance certifications, to address these concerns.
3. Latency
For real-time applications like chatbots, minimizing latency is crucial. Cloud providers offer solutions like edge computing, where computation is performed closer to the end-user, reducing latency.
The Future Of Cloud Infra And Language Models
As advanced language models continue to evolve, so too will the infrastructure supporting them. Here are some future directions:
1. Specialized Hardware
Hardware optimized specifically for AI workloads, such as AI accelerators and neuromorphic chips, will further enhance performance and energy efficiency.
2. Federated Learning
Federated learning enables model training across decentralized devices while preserving data privacy. Cloud infrastructure will play a vital role in orchestrating federated learning workflows.
3. Hybrid Cloud
Hybrid cloud environments, combining on-premises infrastructure with public cloud services, offer flexibility and control, particularly for organizations with regulatory or compliance requirements.
How Hexon Global Can Help With AI and ML on AWS
At Hexon Global, we offer comprehensive support in the following areas:
Strategic AI and ML Guidance
Benefit from our strategic insights and personalized recommendations to design AI and ML solutions that are not only cost-effective but also aligned with your business goals and growth trajectory.
Cost Optimization for AI and ML Workloads
Tap into our deep understanding of AWS pricing structures and optimization techniques to identify cost-saving opportunities, minimize resource wastage, and maximize the return on investment for your AI and ML projects.
Technical Excellence in AI and ML Infrastructure
Count on our team’s technical expertise and hands-on experience in deploying, managing, and fine-tuning AI and ML infrastructures on AWS. We ensure optimal performance and efficiency to meet the demands of your AI and ML workloads.
Make Hexon Global your trusted ally in advancing your AI and ML initiatives on AWS. Reach out to us.
Contact Us
Get in touch
Understand how we help our clients in various situations and how we can be of service to you!